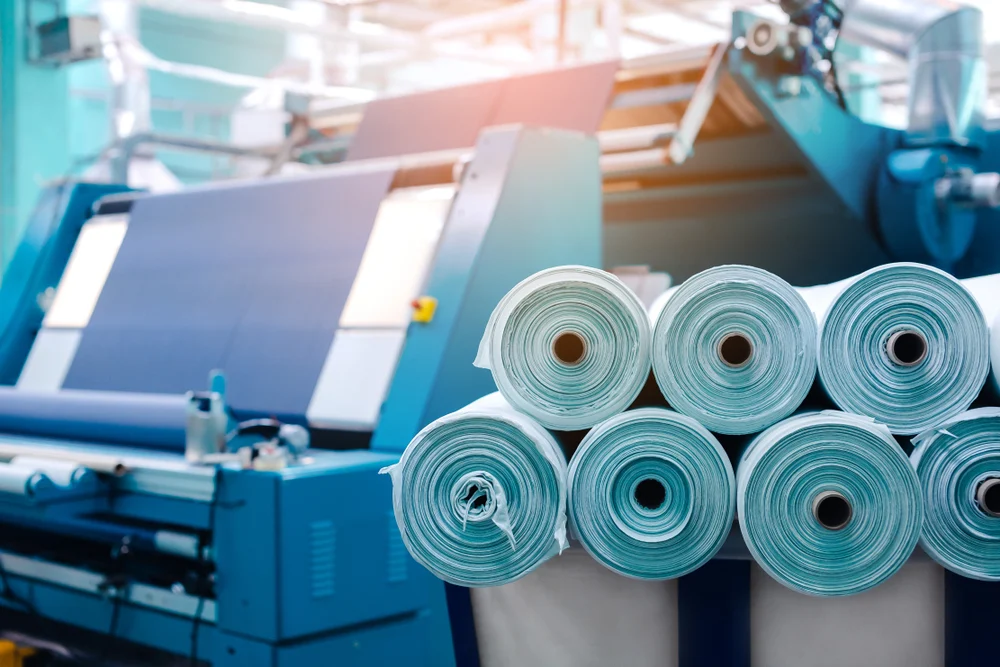
Enhancing Fabric Defect Detection for Everest Textile Co., Ltd.
About the Customer
Everest Textile Co., Ltd. is a prominent textile manufacturer based in Taiwan, renowned for its commitment to high-quality products and sustainable practices. With a reputation for excellence, the company sought to overcome challenges in fabric defect detection to enhance product quality and operational efficiency.
- Company Name: Everest Textile Co., Ltd.
- Industry: Textile Manufacturing
- Headquarters: Taiwan
- Established: 1995
- Core Specializations: Fabric production for apparel, home textiles, and industrial applications
- Mission: To deliver exceptional textile products through innovative technology and sustainable practices.
- Vision: To be a global leader in the textile industry known for quality, innovation, and sustainability.
Challenges
Everest Textile Co., Ltd. faced several significant challenges in their fabric defect detection process:
- High Defect Rates:
- Challenge: The company experienced elevated fabric defect rates, including discoloration, irregular texture, and structural flaws.
- Impact: High defect rates resulted in increased rework, waste, and customer dissatisfaction, affecting overall product quality and operational costs.
- Manual Inspection Process:
- Challenge: Fabric inspection was performed manually by quality control inspectors who visually checked for defects.
- Impact: The manual process was time-consuming, prone to human error, and inconsistent, leading to missed defects and quality issues.
- Inefficiency in Defect Identification:
- Challenge: The existing process lacked the capability to swiftly and accurately identify and classify defects.
- Impact: Inefficient defect identification led to delays, increased production costs, and reduced customer satisfaction.
- Limited Automation:
- Challenge: There was minimal automation in the defect detection process, limiting scalability and consistent quality maintenance.
- Impact: The lack of automation contributed to slower production cycles and higher operational costs.
Project Objectives
To address these challenges, the following objectives were defined for the project:
- Develop an Advanced Fabric Defect Detection System:
- Objective: Implement a sophisticated solution using machine learning and computer vision technologies to automate and enhance defect detection.
- Implement Real-Time Defect Detection:
- Objective: Enable real-time analysis of fabric rolls to promptly identify and classify defects.
- Improve Quality Control:
- Objective: Enhance quality control processes through automation and data-driven insights.
- Streamline Operations:
- Objective: Automate workflows and provide actionable insights to improve overall operational efficiency.
Approach
Flivo AI employed the following approach to develop and implement the fabric defect detection system:
- Data Consolidation:
- Action: Collected and integrated fabric images and defect data into a centralized repository.
- Goal: Provide comprehensive visibility into fabric quality and defect patterns.
- Machine Learning Model Development:
- Action: Developed a custom machine learning model to identify and classify various fabric defects.
- Goal: Enhance accuracy in defect detection and reduce manual inspection errors.
- Computer Vision Integration:
- Action: Installed high-resolution cameras and integrated computer vision technology to capture and analyze fabric surfaces in real-time.
- Goal: Automate defect detection and classification during production.
- Automated Defect Detection System:
- Action: Implemented an automated system for real-time defect analysis, including alerts and severity classification.
- Goal: Improve defect identification speed and accuracy.
- Quality Control Dashboard:
- Action: Developed a user-friendly dashboard to monitor defect detection results and generate reports.
- Goal: Provide real-time insights and facilitate data-driven decision-making.
- Training and Support:
- Action: Provided training to the quality control team and ongoing technical support.
- Goal: Ensure effective use of the new system and address any issues promptly.
Project Highlights
- Timeline: 12 weeks (approximately 3 months) from project kickoff to application production.
- Team: A small, agile team of app developers and data engineers.
- Scope: Initial implementation at 7 global sites.
- Data Volume: Monitored and analyzed data from 80,000 suppliers and 3 million item details.
- Integration: Data from 10 different systems, including common ERPs.
Results
reduction opportunity across sourcing costs.
global facilities implemented the system
Significant improvements in defect detection
Benefits
- Improved Product Quality:
- Benefit: Enhanced defect detection accuracy, leading to higher product quality and reduced defect rates.
- Increased Operational Efficiency:
- Benefit: Streamlined defect detection process through automation, reducing inspection time and costs.
- Enhanced Quality Control:
- Benefit: Better oversight and control over fabric quality through real-time monitoring and actionable insights.
- Competitive Advantage:
- Benefit: Strengthened market position by adopting advanced technology and improving product quality.
Proven results in weeks, not years
Exec. Briefing
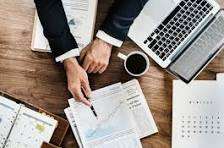
Exec. Briefing
2 Hours
Technology
Assessment
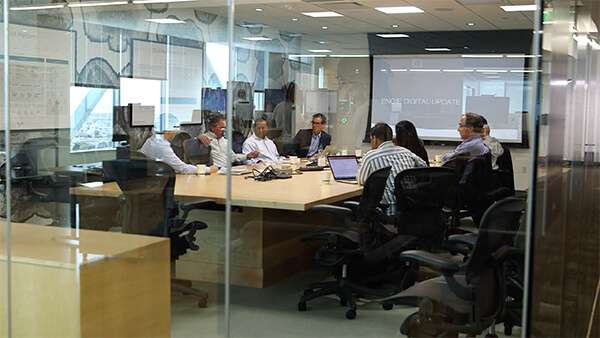
Technology
Assessment
2-3 Days
Production
Trial
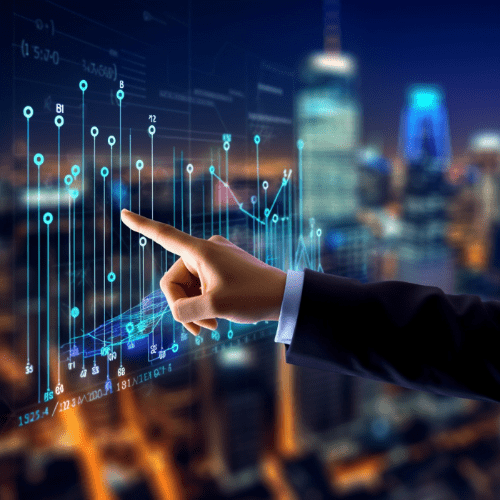
Production
Trial
8-12 Weeks
AI Application
Deployment in Production
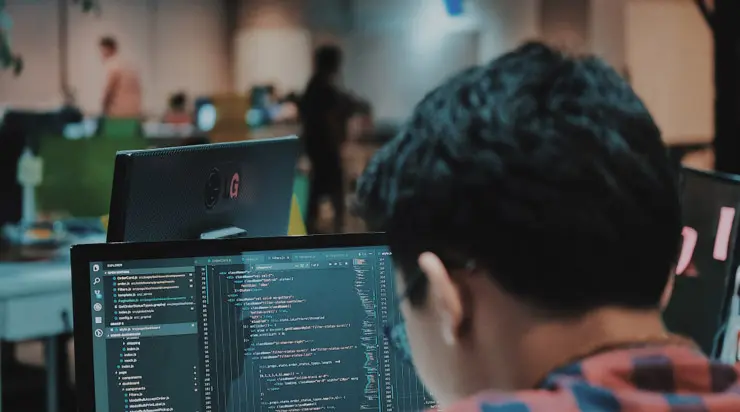
AI Application
Deployment in Production
3-6 Months