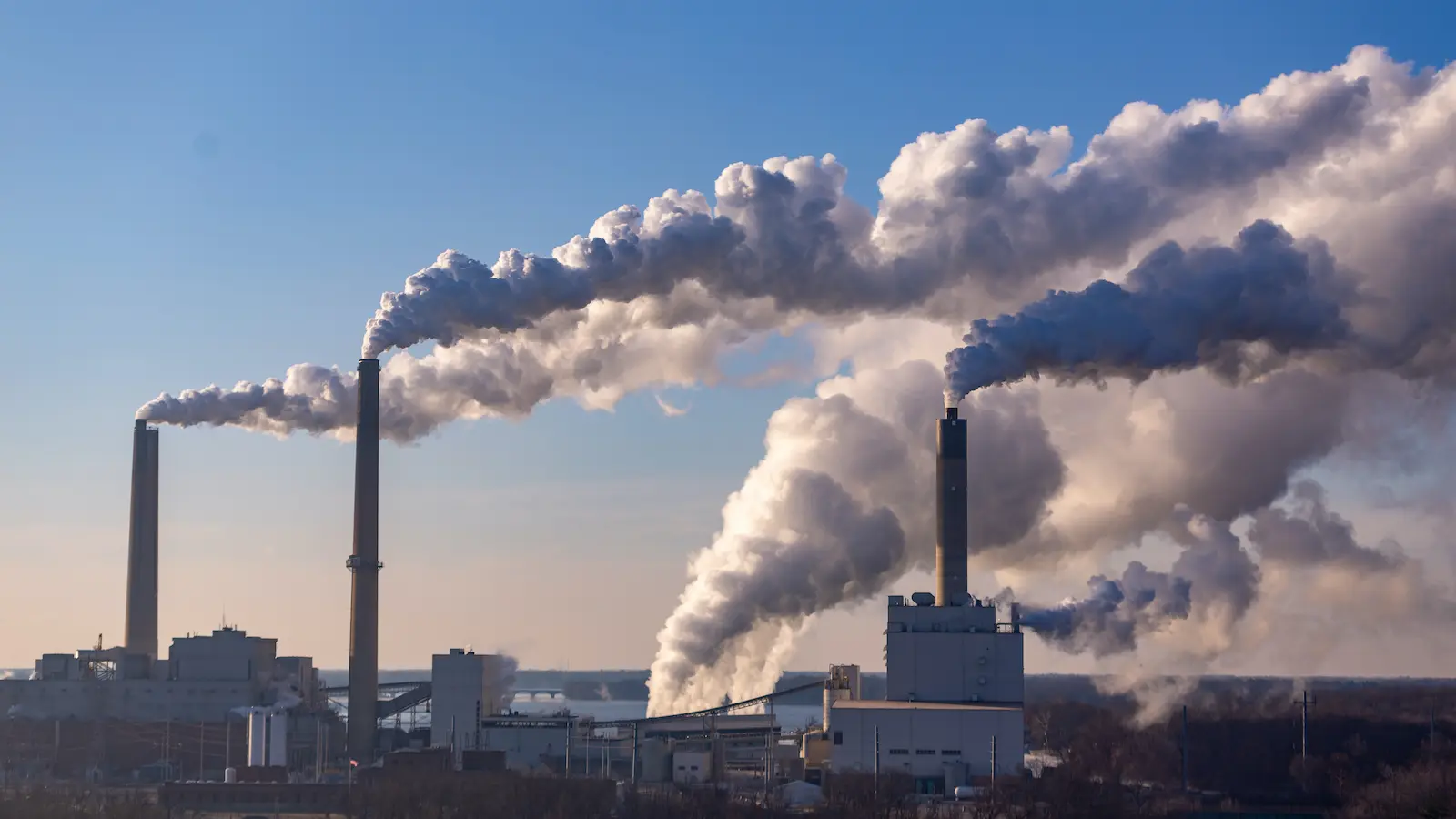
Optimizing Energy Production and Efficiency with Predictive Modeling
About the Customer
This Flivo AI customer is a leading renewable energy provider in China, specializing in large-scale solar and wind energy production. The company serves over 50 million households and operates across Eastern China. As a result of unpredictable weather and fluctuating demand, the company needed a solution to improve energy production and distribution.
- Industry: Renewable Energy
- Location: China
- Key Challenges: Unpredictable weather, fluctuating energy demand, energy wastage, and grid overloads.
Challenges
The customer was grappling with several key issues in managing their energy production and distribution:
- Unpredictable Weather: Relying on solar and wind energy made the company vulnerable to weather fluctuations, leading to a 20% deviation from forecasts, which affected supply reliability.
- Fluctuating Energy Demand: Rapid urban growth and changing economic conditions caused a 15% mismatch between energy production and demand, resulting in inefficiencies and wasted resources.
- Energy Wastage & Grid Overloads: Off-peak production led to 18% energy wastage, costing the company 500 million RMB annually. Additionally, peak periods caused frequent grid overloads, leading to blackouts and costing nearly 1 billion RMB annually in backup power generation.
Project Objectives
The primary goals of the project were:
- Accurate Energy Demand Forecasting: Develop a predictive model to align energy production with real-time demand to reduce wastage and optimize production.
- Minimize Grid Overloads: Implement solutions that predict and mitigate grid overloads, reducing the need for costly emergency power.
- Cost Reduction: Lower operational costs by improving production efficiency and minimizing energy wastage.
- Sustainability Goals: Reduce the company’s reliance on fossil fuels for backup power, improving its sustainability profile and meeting regulatory standards.
Approach
Flivo AI designed and implemented a multi-model predictive analytics solution, using machine learning and time-series forecasting techniques. The key steps involved:
- Data Collection & Preprocessing: Flivo AI gathered three years of data on energy production, consumption, and weather conditions. Data was cleaned, normalized, and preprocessed to ensure accuracy.
- Predictive Modeling: The solution used a combination of forecasting models:
- Prophet: To capture long-term trends and seasonality for energy demand.
- ARIMA (AutoRegressive Integrated Moving Average): For day-to-day short-term demand forecasting.
- LSTM (Long Short-Term Memory): A deep learning model to handle non-linear relationships between variables such as weather and demand.
- Random Forest: To integrate external factors like weather forecasts and time of day into the predictions.
- Prophet: To capture long-term trends and seasonality for energy demand.
- Model Evaluation & Implementation: Flivo AI trained and evaluated each model using metrics like RMSE (Root Mean Squared Error) to ensure the highest accuracy before deploying them for real-time energy production optimization.
Project Highlights
- Implementation Timeline: The solution was developed and deployed over the course of several months, integrating historical data and real-time updates.
- Technology Stack: Time-series forecasting models like Prophet and ARIMA were combined with machine learning techniques (LSTM, Random Forest) to deliver a comprehensive, multi-faceted predictive solution.
- Key Areas Addressed: Energy demand forecasting, grid overload prevention, energy efficiency improvement, and cost reduction.
Results
Energy Efficiency Improved
Grid Overload Reduction
Cost Savings Anually
Metric Tons of Carbon Emission reduced Annually
Benefits
- 25% Improvement in Energy Efficiency: The predictive models aligned energy production with demand, reducing energy wastage from 18% to 7%, saving 400 million RMB annually.
- 15% Reduction in Grid Overloads: By predicting peak periods, the company reduced grid overloads, saving 600 million RMB in emergency power costs.
- 15% Reduction in Costs: Optimizing energy production lowered the company’s reliance on expensive backup power, resulting in overall savings of 1.2 billion RMB annually.
- Environmental Impact: The reduction in energy wastage contributed to a 10,000 metric ton reduction in greenhouse gas emissions annually, improving the company’s sustainability profile.
Proven results in weeks, not years
Exec. Briefing
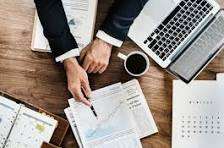
Exec. Briefing
2 Hours
Technology
Assessment
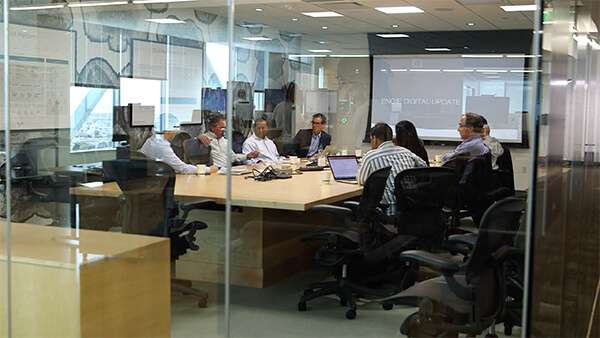
Technology
Assessment
2-3 Days
Production
Trial
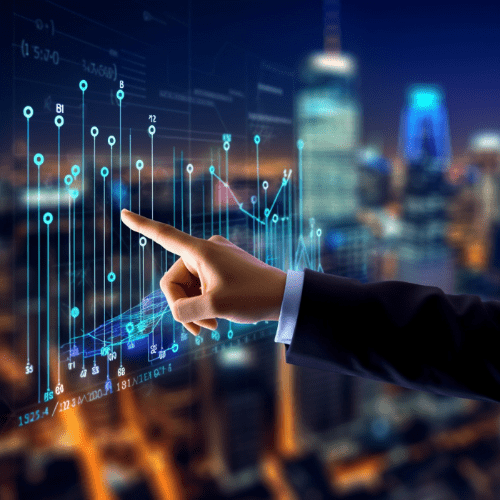
Production
Trial
8-12 Weeks
AI Application
Deployment in Production
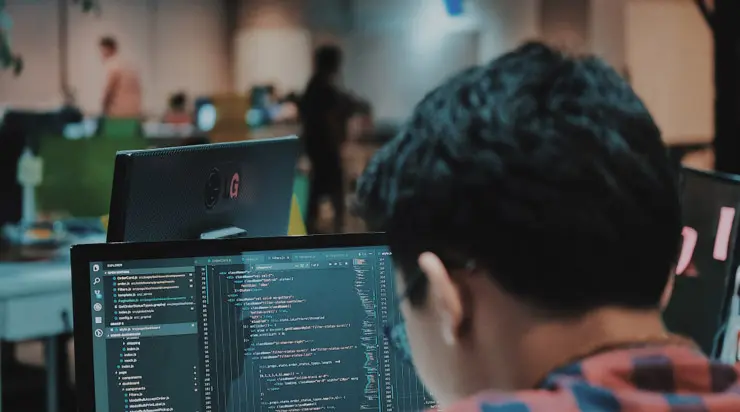
AI Application
Deployment in Production
3-6 Months